
Likes
School of Physics and Telecom Engineering, with the Frontier Research Institute and the Guangdong Provincial Key Laboratory of Quantum Engineering and Quantum Materials gained ground in quantum machine learning by publishing their research findings under the title Protocol for implementing quantum nonparametric learning with trapped ions in Physical Review Letters (PRL), one of the most prestigious peer-reviewed journals in the field of physics. The research team initially discovered quantum computers’ greater ability in learning from existing experience.
Humans are good at learning from experience and predicting new situations by comparing them to situations in the past. In machine learning, this approach is known as "Nonparametric Learning." Through Nonparametric Learning we are able to make reliable predictions by extracting information from similarities between a new set of input data and all samples. If a quantum computer were capable of learning, would it be good at learning from “experience” (Data)? Would it display stronger learning ability (display quantum advantage)? If the answer is “yes”, then how? In view of the above questions, the quantum AI research team of the Frontier Research Institute proposed the quantum version of "Nonparametric machine learning" and designed an intelligent trapped ion quantum computer.
In the findings, the data set is innovatively loaded as superposition quantum state and the entanglement structure of quantum state is applied to design the quantum algorithm, which helps efficiently process information and send further predictions. In the new approach, by encoding data into quantum feature space at the same time, this brings not only quantum acceleration, but also a stronger descriptive ability for the “expression” of data. Researchers expect that under the new scheme quantum advantages will be apparent in dealing with quantum machine learning problems and solving scientific problems, such as drug molecular design.
Postdoctoral fellow Zhang Danbo is the first author of this research paper, while Professor Zhu Shiliang and Professor Wang Zidan from the university of Hong Kong are co-corresponding authors. The research was funded by the National key R&D program, the National Natural Science Foundation (NSFC), the Guangdong Provincial Research Plan in Key Areas and the Guangdong Provincial Natural Science Fund, etc.
Paper Links
https://link.aps.org/doi/10.1103/PhysRevLett.124.010506
Source from News Center
Translated by: Ma Kaiyu
Proofread by: Edwin Baak
Reviewed by: Li Jianru
What to read next:

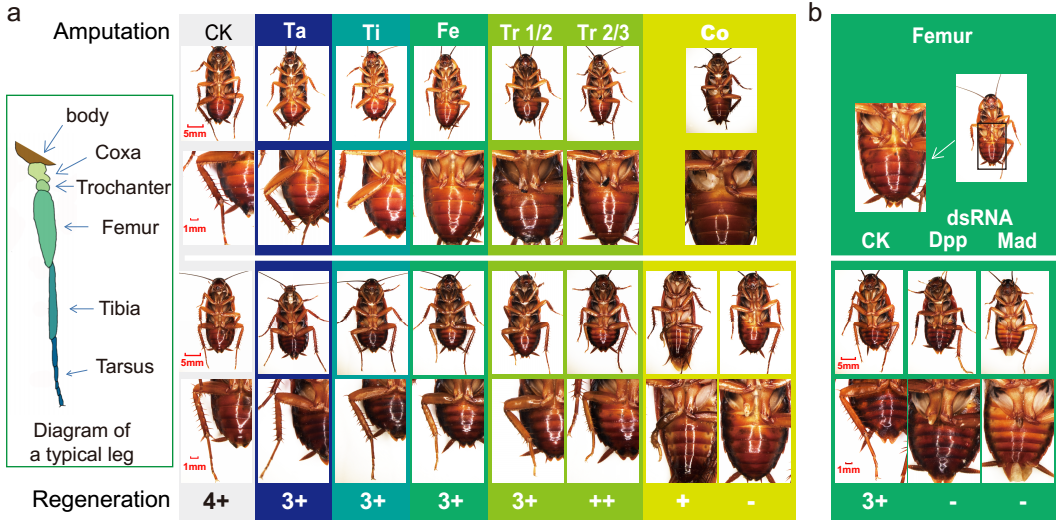
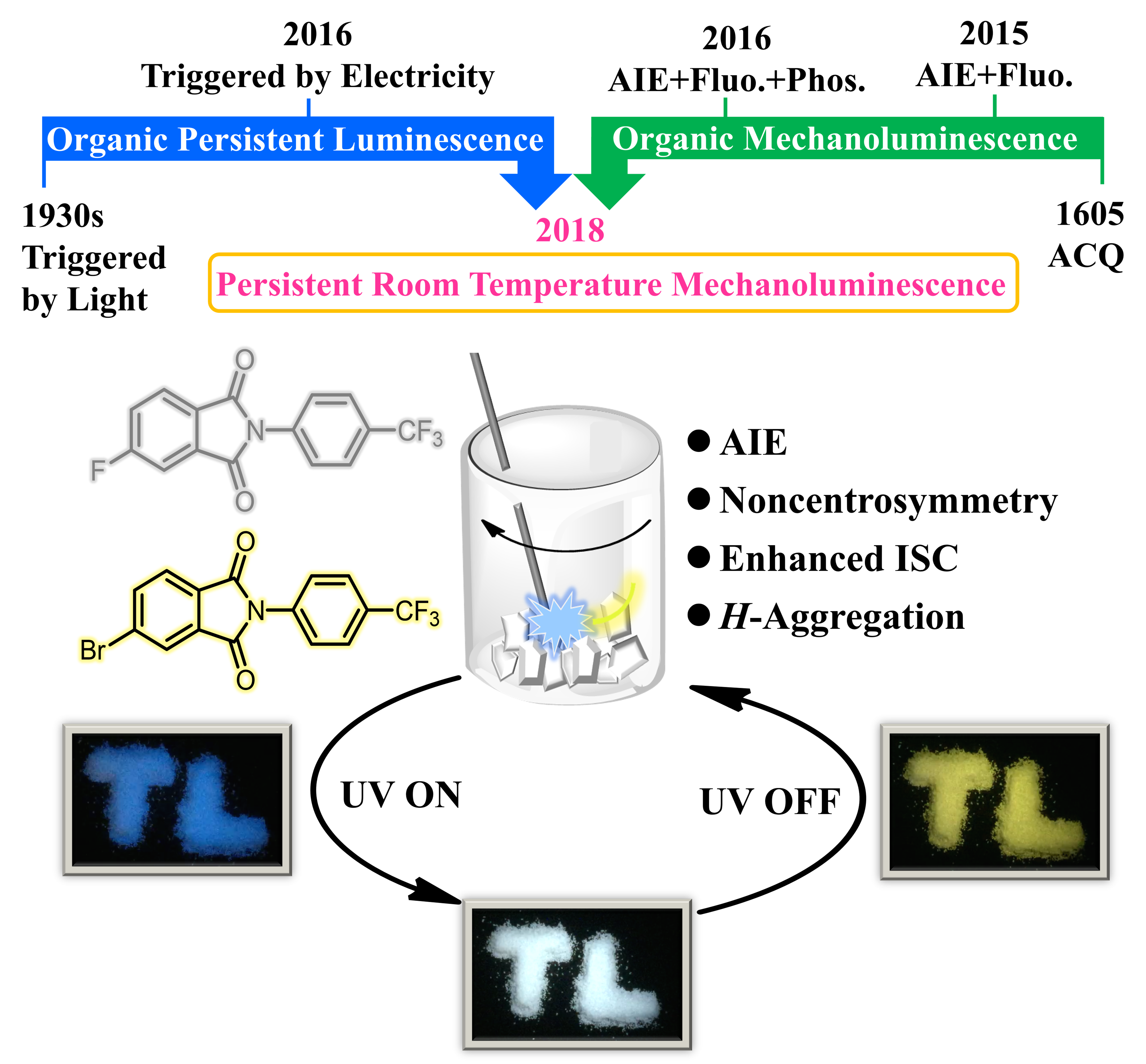
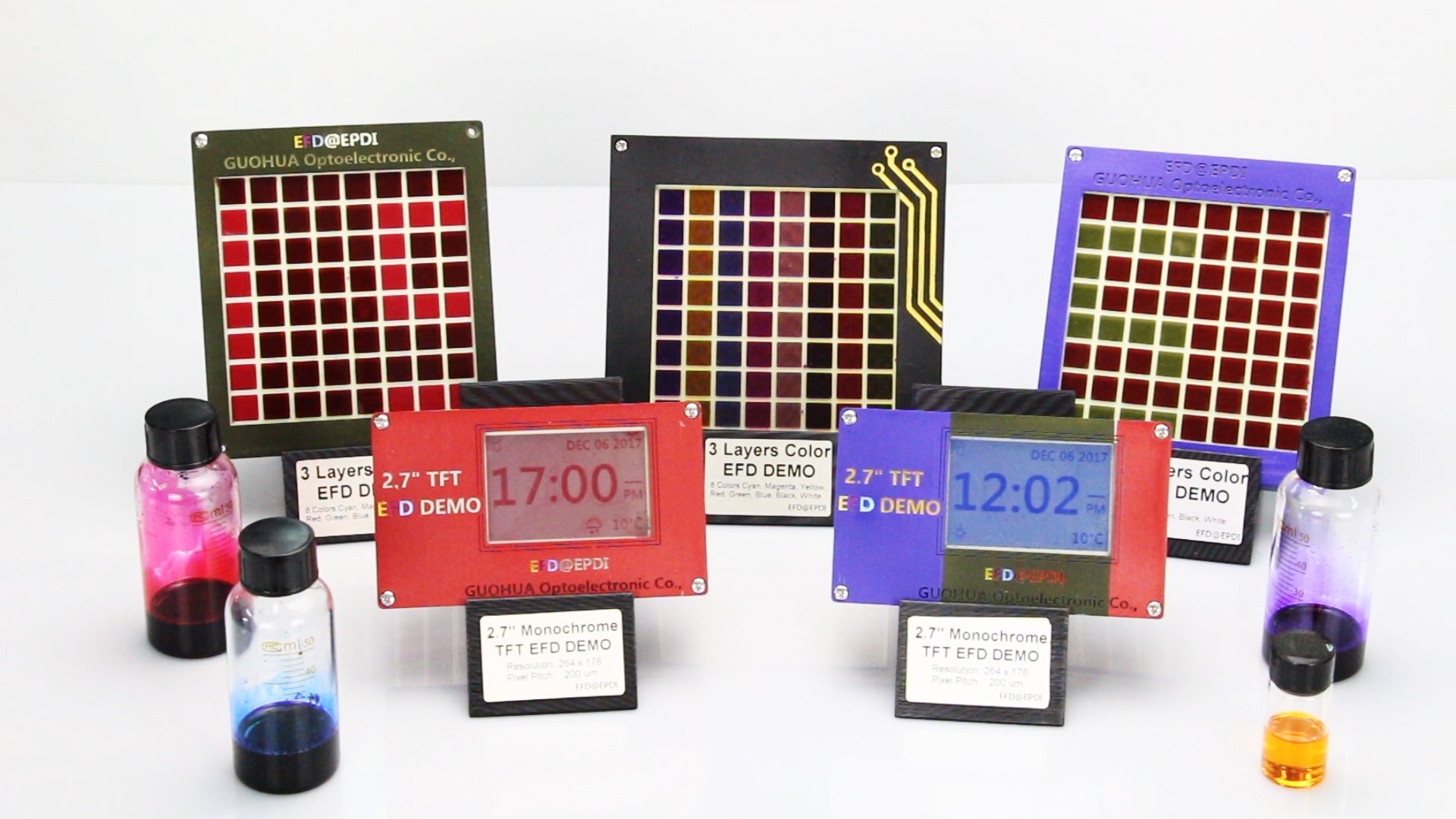
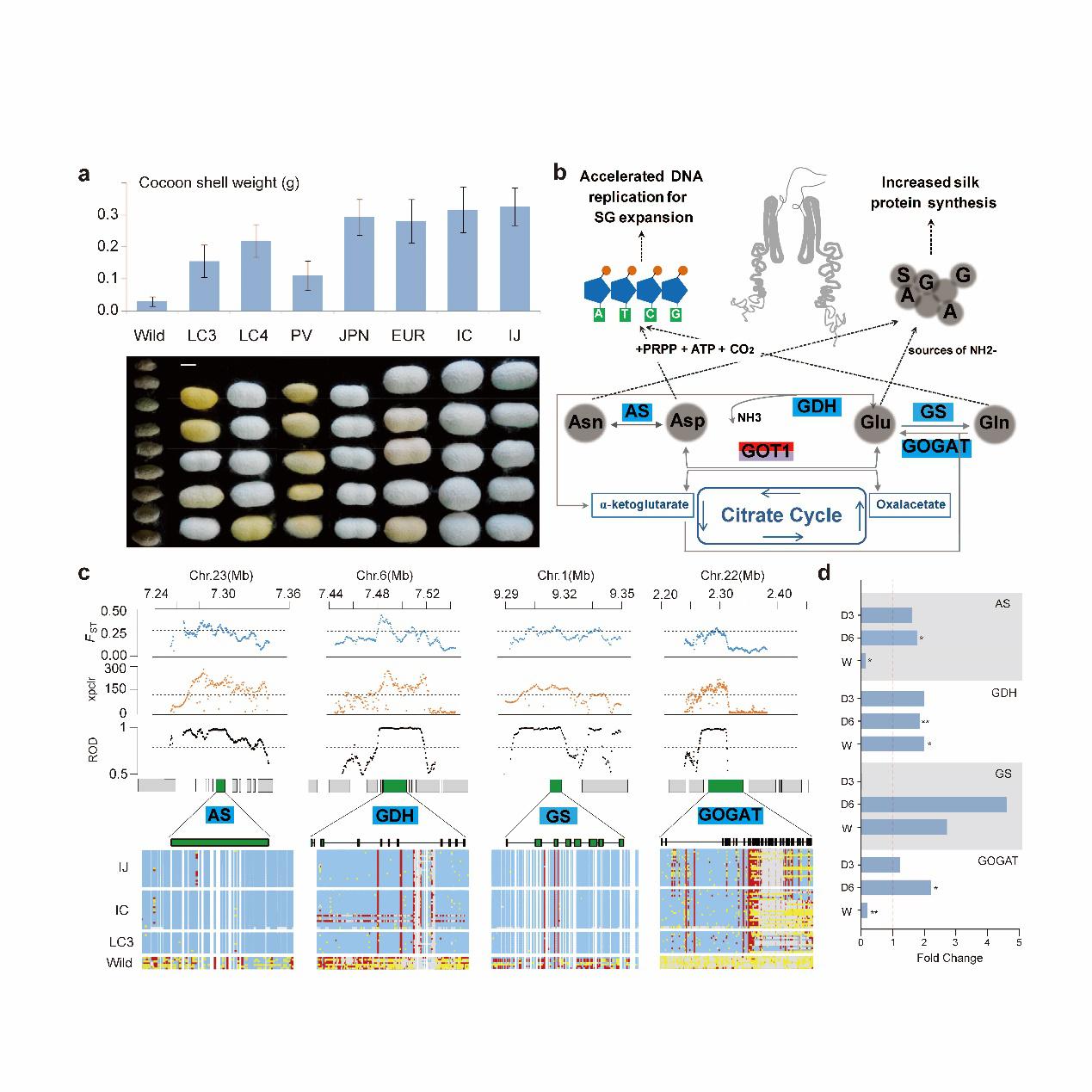
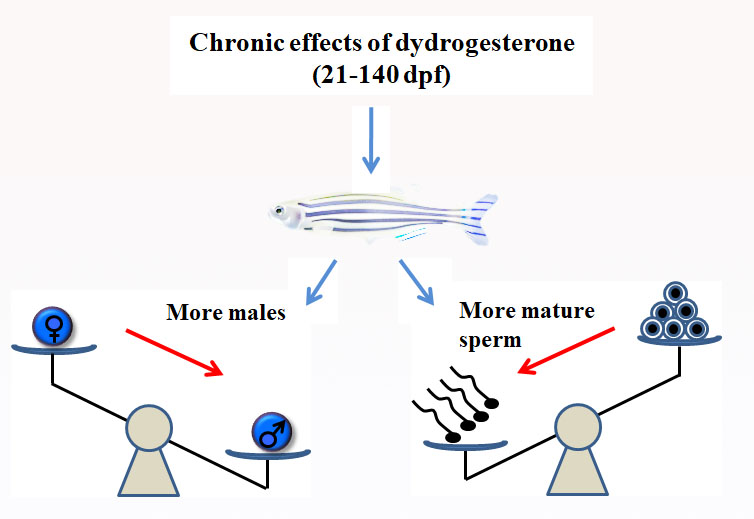
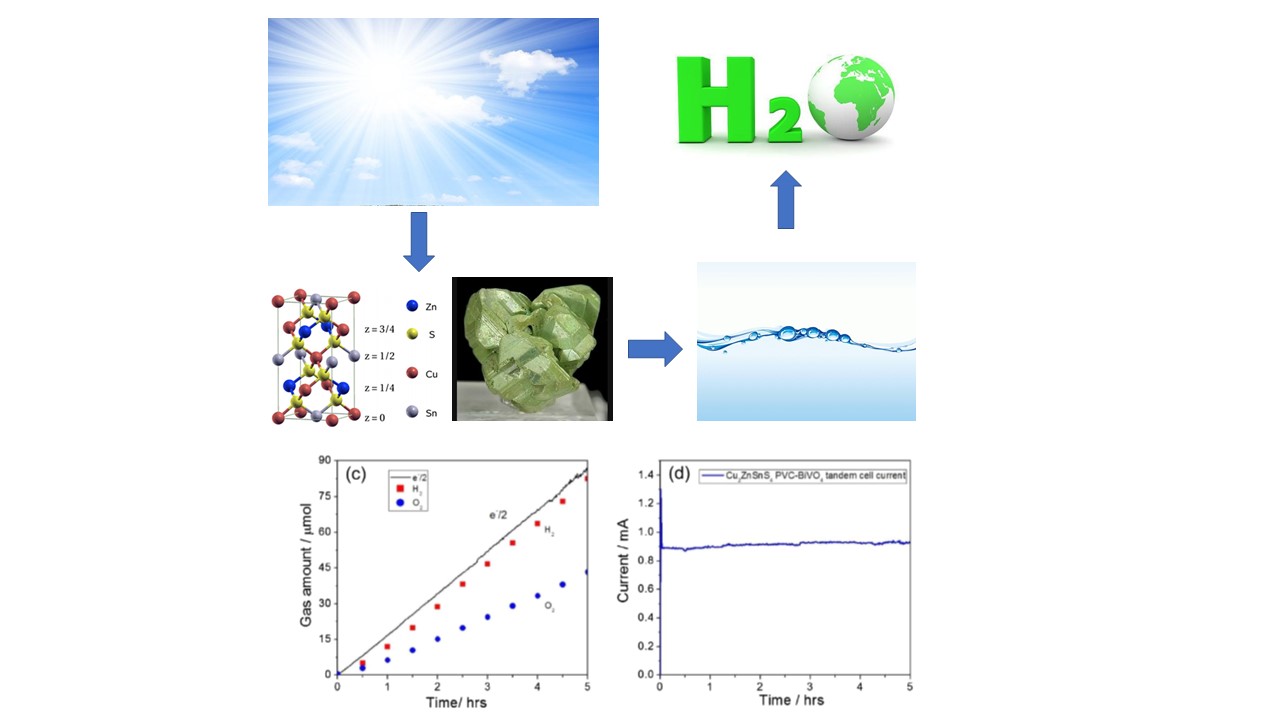
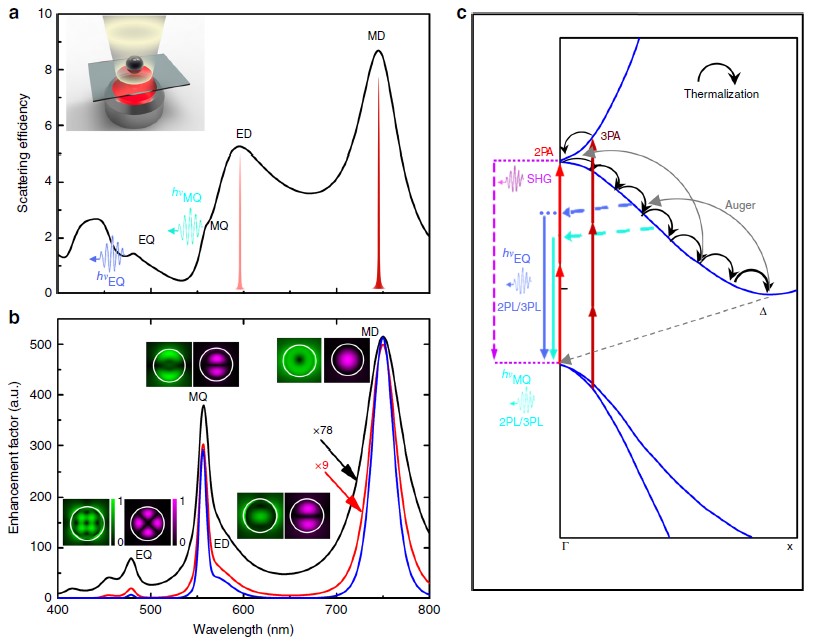
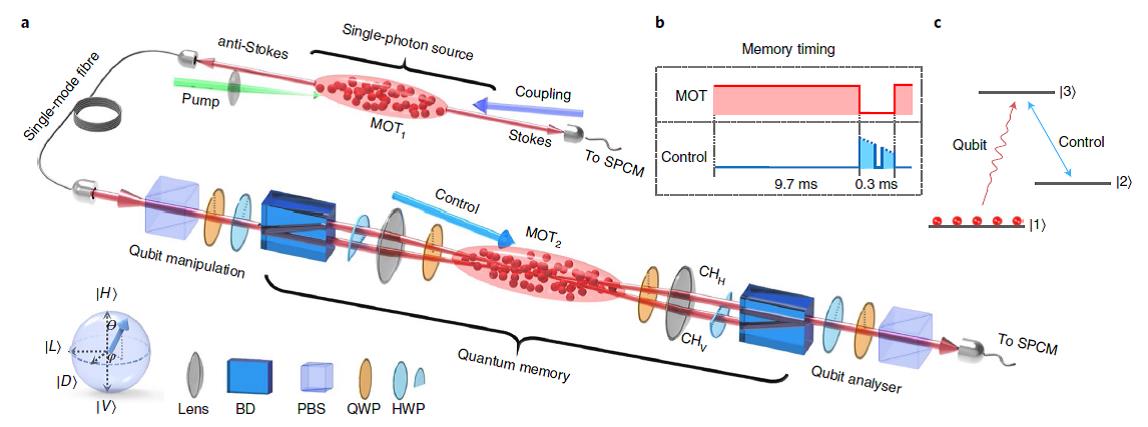
